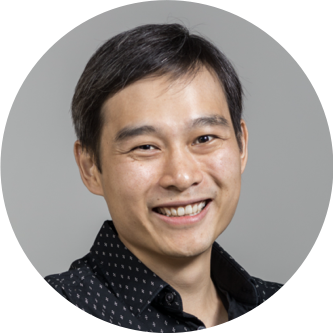
Hello, I'm Harold.
I’m an Assistant Professor of Computer Science at the School of Computing, National University of Singapore (NUS). I direct the Collaborative, Learning, and Adaptive Robots (CLeAR) Lab. My research interests are in broadly in human-AI/robot interaction, machine learning, and robotics. I’m particularly interested in developing trustworthy collaborative robots. Read more about my research, teaching or get in touch.
Latest News
27 Mar 2024
Excited to be giving a Keynote at ICRA'24! I'll be speaking about our lab's work on generative models for robotics.
22 Sep 2023
Both our submitted papers were accepted to Neurips 2023. Check out our spotlight paper on making deep generative models forget.
30 Aug 2023
CLeAR has three papers at IROS 2023. Come join us at IROS!
1 Jun 2023
Honored to be selected for an Early Career Spotlight talk at RSS 2023!
25 Apr 2023
Our work on a family of stable and effective continuous-discrete state-space models was accepted to ICML'23.